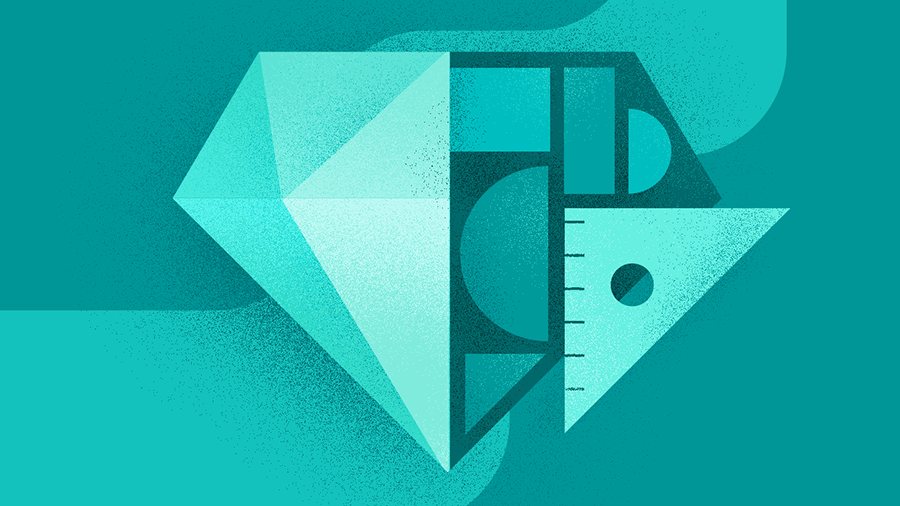
Autorzy
Szczegóły publikacji
Streszczenie
Streszczenie publikacji
Abstract
In this paper, we propose a method for rules generation for images, which can be further used to enhance the classification accuracy of a convolutional neural network as well as to increase the level of explainability of neural network decisions. For each image from the training set, a descriptor is created based on the information contained in the convolutional layers of the network. The descriptors of images of the same class are then used to create a single-class prototype, which is characteristic of that class. The prototypes of different but semantically similar prototypes are then grouped into major categories using the DBSCAN algorithm. The analysis is carried out using the ILSVRC dataset and the VGG16 net is used. The experimental verification performed on the validation set demonstrated the validity and general nature of the rules obtained using the proposed method.